What Are the Data Quality Standards?
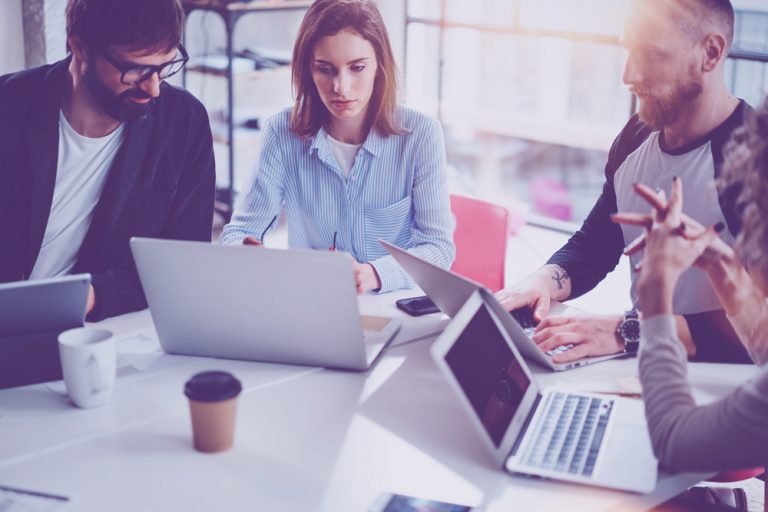
What Are the Data Quality Standards?
Data quality is an important consideration for all organizations regardless of size or industry. The quality of data can affect the success of a business in a number of ways. Standards for data quality involve a set of guidelines that organizations use to ensure that their data is accurate, consistent, and reliable. Keep reading to learn more about data quality standards and how they can benefit your organization.
Table of Contents
The Standards of Data Quality and Information Governance
Information governance (IG) is a framework of policies and procedures that organizations put in place to ensure the quality of their data. Quality data is important for two reasons. First, it enables organizations to make better decisions since quality data is more accurate and reliable. Second, it protects the organization from legal and financial risks associated with poor data quality. There are a number of factors that contribute to standards of good data. The most important standards are accuracy, completeness, timeliness, and consistency. Accuracy means that the data is correct and up-to-date. Completeness means that all relevant information has been included. Timeliness means that the data is current. And consistency means that the same rules are applied to all instances of the data. To ensure the quality of data, organizations should establish clear standards for what qualifies as good data and implement processes to verify that the quality meets these standards. They should also have a plan for dealing with bad or inaccurate data when it does occur. This may include techniques like cleansing and scrubbing, which remove erroneous entries from databases or other repositories.
Benefits of Data Quality
The benefits of data quality are vast and far-reaching for businesses. Poor data quality can cause business problems such as inaccurate reports, incorrect decisions, and missed opportunities. However, when data is of good quality, businesses can achieve significant improvements in efficiency, accuracy, and customer satisfaction. Some of the key benefits of data quality include improved efficiency, accurate reports, improved customer satisfaction, and reduced business risks. When data is of good quality, businesses can reduce the time needed to make decisions and complete tasks. This improved efficiency can lead to significant cost savings and improved competitiveness. Good quality data leads to accurate reports, which in turn allows businesses to make better decisions based on accurate information. Businesses can also provide more accurate and consistent information to their customers. This improved customer service leads to increased customer satisfaction and loyalty. Poor data quality can lead to a number of business risks, such as missed opportunities, incorrect decisions, and inaccurate reports. High-quality data helps businesses avoid these risks, and leads to improved decision-making and a more successful business.
Understanding Business Rules and Their Impact on Data Quality
It is important to ensure data quality because it can impact business decisions and operations. Business rules are statements that define how data should be interpreted and used in order to achieve specific results. They are used to govern the collection, entry, storage, retrieval, and use of data. Business rules can also help improve data quality by ensuring that data is consistent and accurate. They also help ensure that information is used correctly so that it achieves the desired results. Improving data quality can help businesses improve their operations.
The Challenges of Data Quality Management
There are several challenges that organizations face when trying to manage data quality. The first is the sheer volume of data that needs to be managed. Organizations today are collecting and storing more data than ever before. The second challenge is the variety of data formats and sources. Data can come from internal and external sources, in a variety of formats, such as text, numbers, images, and video. The third challenge is the rate of change. Data can change rapidly, and it can be difficult to keep up with the changes. The fourth challenge is the lack of standardization. There is no single definition of data quality and no standard method for measuring it. The fifth challenge is the lack of clarity about who is responsible for data quality. Many organizations struggle to determine who is responsible for ensuring the quality of data.
There are several strategies that organizations can use to manage data quality. The first is data cleansing. This involves identifying and correcting inaccurate or incomplete data. The second is data validation. This involves verifying the accuracy and completeness of data. The third is data governance. This involves setting standards for data quality and developing policies and procedures for ensuring that data meets these standards. The fourth is data integration. This involves combining data from multiple sources into a single, cohesive data set. The fifth is data mining. This involves extracting valuable information from large data sets. Despite the challenges, data quality management is essential for organizations today. Poor data quality can lead to significant financial losses, so it is important to take steps to ensure the quality of your data. Organizations that take data quality seriously are more likely to make accurate business decisions, which can lead to increased profits and competitive advantage.
How to Identify and Correct Data Quality Issues
There are many different ways to identify and correct data quality issues. One common method is to use a data quality assessment tool. This tool can help you locate the specific areas of your data that need improvement. Another method is to use business rules. Business rules are a set of guidelines that help you determine whether or not your data is of high quality. You can also use sampling techniques to identify and correct data quality issues. Sampling involves selecting a small portion of your data and analyzing it in order to determine its quality. Finally, you can use verification and validation techniques to ensure the accuracy of your data. Verification involves checking the results of your analysis against some sort of reference point. Validation involves ensuring that the data meets certain requirements before it is used for decision-making purposes.
Overall, quality standards are important because they ensure that data is reliable and accurate. This is important for organizations because it allows them to make informed decisions based on accurate data.
Lucas Noah, a distinguished writer with a Bachelor of Information Technology (BIT) degree, is currently making waves in the digital content sphere with his contributions to Creative Outrank LLC and Oceana Express LLC. His work on their websites showcases hi... Read more